Demystifying Artificial Intelligence
Amid all the hype, there is significant commercial activity underway in the area of AI that is affecting organisations in every sector. Business leaders should understand what AI really is and where it is heading. Join us on a journey through the AI revolution with our exclusive three-part series. Discover what AI means for our community. In part one of our three part series we even the playing field and demystify AI.
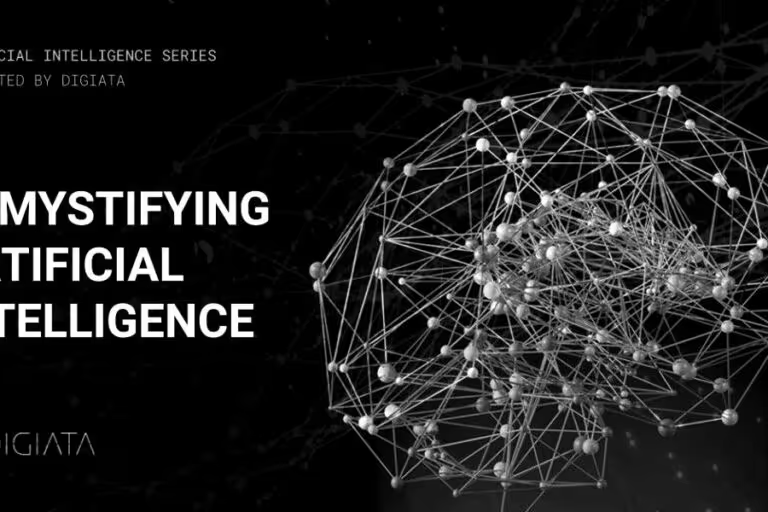
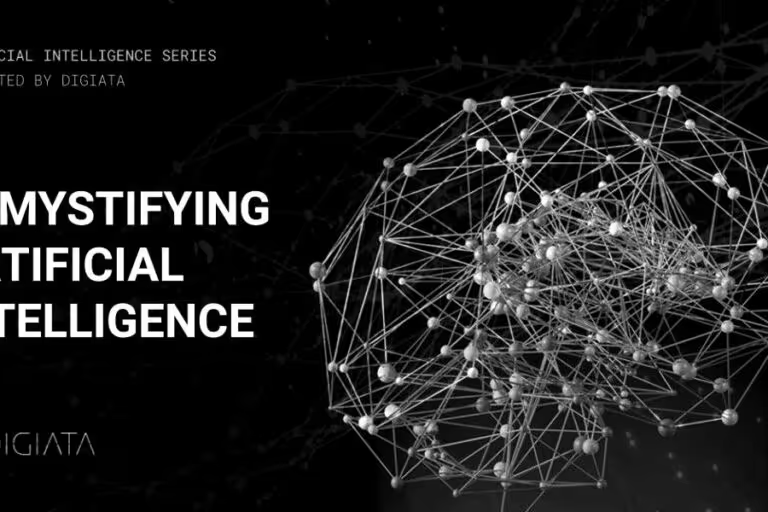
What is Artificial Intelligence?
Artificial intelligence is very much at the forefront of almost all aspects of our everyday lives and the truth is that it’s been there for much longer than we’d assume. Artificial Intelligence involves the designing of technology with the purpose of being fully independent and adaptive in its applications. There are 3 generally accepted stages of Artificial Intelligence each defined by the capacity of the application to excel in multiple fields of knowledge: Artificial Narrow Intelligence (ANI), Artificial General Intelligence (AGI) and Artificial Super Intelligence (ASI).
What is Artificial Narrow Intelligence?
Artificial Narrow Intelligence (ANI) also referred to as Weak AI is AI designed with a very specific knowledge field and application in mind. Machine Learning (ML) would fall under this AI variant. A common misconception exists, namely that Machine Learning and Artificial Intelligence exist as separate entities. This however is not the case. Artificial Intelligence is the overarching umbrella term under which Machine Learning is a subset. Artificial Intelligence is characterised by its aim of mimicking (and eventually surpassing) human capability. We often make the mistake of envisioning this ‘human capability’ as a genius level intelligence in a variety of fields, however human intelligence comprises a spectrum of capabilities and modes. We have ‘auto-pilot’ mode that we use when doing repetitive and mundane tasks. In this mode we’re not thinking creatively or re-imagining a solution to a problem, we’re just accomplishing the task the way our boss asked us to, nothing more, nothing less. We also have ‘creative modes’ when we’re innovating and approaching problems in unique ways. We even have ‘delusional modes’ where we act on misinformation or misunderstanding. Human intelligence cannot be pigeon-holed/contained into a single connotation or description and this should also apply to Artificial Intelligence.
What is Machine Learning?
Machine Learning is the ‘auto-pilot mode’ of Artificial Intelligence where a machine ‘learns’ how to provide the most accurate solution to problems given to it by using test data, it is not required to think out of the box or provide recommendations on new ways to approach a problem. There is a defined solution method, the success of its implementation depends on how accurately and efficiently it can perform using the defined solution method. It differs from traditional programming in the sense that it is designed to adjust its learning model based on data fed to it and improve its accuracy every iteration. There is no need for constant human intervention to adjust machine learning models. These models ‘learn’ in 4 main ways:
- Supervised Learning: the model is given examples of how it should classify data points
- Unsupervised Learning: the model is given data points with no desired classification and it makes inferences based on data point characteristics
- Semi-supervised Learning: a mix of the two previous types
- Reinforced Learning: the model learns what is expected in real time by receiving feedback
Most often when Artificial Intelligence and Machine Learning are seen as separate fields it stems from the misconception that Artificial Intelligence is only the multi-faceted machine capable of contextualising and answering questions using various sources based on data presented to it. This is only one stage of Artificial Intelligence called Artificial General Intelligence (AGI).
What is Artificial General Intelligence?
AGI is one of the stronger forms (Artificial Super Intelligence ASI is currently only theoretical) of AI and it is capable of independent thought and also able to discover new information, make decisions by rationalising and contextualising the data presented. AGIs such as OpenAI’s ChatGPT, Google’s Gemini or IBM’s Watson are designed to perform on par with human capability (ASI would surpass human capability). AGIs surpass ANIs as they have a broader range of capabilities and knowledge to apply in their applications. In order for AGIs to fulfil their capabilities they need to understand and communicate in Natural language. They do this by using statistical models to interpret human language and output their results in the same form (Natural Language Processing NLP). This is the technology behind chatbots like ChatGPT, Siri, Alexa, and others. Large Language Models (LLMs) are a subfield of NLP and are used for predicting the next word in a sequence based on the preceding words. LLMs basically use probabilities for sentence structures to generate text in comprehensible human language. Beyond understanding language AGIs can also interpret their visual information from their surroundings (Computer Vision) e.g. self-driving cars can ‘see’ traffic. In order to provide the highest quality outputs AGIs are required to perform at expert levels in the fields/domains they are assigned (domain specific mastery systems). This area involves the use of explicitly stated rules and knowledge bases in an attempt to imitate the decision-making of an expert in a certain field. It is the field behind Creatio’s Copilot AI which allows it to efficiently offer solutions in the fields of Sales, Marketing, Commerce and Customer Service with a high level of expertise for each. Using the above mentioned fields in conjunction allows AI systems to perceive, process, and act in the physical world (robotics). Adding on to Computer Vision, Robotics allow the AI to contextualise consequences of real-world object interaction through algorithms.
Machine learning is the component of the AI field that is focused on accomplishing tasks specific to a certain field and in a defined manner. The model is trained on data it would be expected to work on and the more relevant the data is to the desired task the more accurate and reliable the model would be. Machine learning works in applications where there are strong rules and regulations and the tasks being accomplished are more repetitive in nature and have clear ‘right’ and ‘wrong’ ways, example would be an auditing algorithm in the world of accounting where there are specific standards (e.g. GAAP) that firms are expected to follow. Even though there may be different ways of representing financial data, having a universally agreed upon standard is mandatory to allow for transparency and comparisons to be made concerning performance of different companies. Comparing the more task and process focused machine learning application to a ‘free’ and innovative general AI would not be ideal for applications where things need to be done a specific way. A more general AI would thrive in environments where innovation is encouraged and where a firm is open to the AI solving a solution in a different way to the ones they are used to. This AI would be ideal in a situation where the problems posed to it are not specific and could originate from any number of varying fields of knowledge as the more capable AI would be adaptable enough in its learning, understanding and reasoning when coming up with solutions.
To summarise the difference in approach when looking at Machine Learning compared to higher level AI application: AI is a tool designed to handle a broad scope of problems mimicking human capability that goes for the optimal solution to a problem while Machine learning is a domain specific look designed to optimise a specified solution method to a specific problem.
This article is part of a three-part series, continue through the rest of the series to find out more about AI and how Digiata can leverage it to unlock value for our clients: